Deepfake Detection: Separating Fact From Fiction
In 2022, Vitali Klitschko appeared to video call German, Austrian, and Spanish politicians regarding the Ukraine war. However, what seemed like a genuine conversation turned out to be a deepfake prank. It took a few minutes for the mayors of Madrid and Berlin to catch on. Officials in Vienna were slower to realize they were speaking to fake content.
A deepfake refers to the “manipulation or artificial generation of audio, video, or other digital content to make it seem like a certain event occurred, or that someone behaved or looked differently than reality,” according to the European Data Protection Supervisor. The Klitschko case might feel like a far-removed example from your everyday life. But deepfake technology and accuracy is developing rapidly. Before you know it, a conversation with your manager or coworker over Zoom could be a deepfake.
So with deep learning tech advancing rapidly, how can we distinguish fact from fiction? Is it possible to detect deepfakes? The ultimate question for us as investors working with founders in this space is: Can technology provide an effective solution to deepfake threats?
To better understand the possibilities, we spoke with three experts specializing in everything from empirical communication to cybersecurity to find out where the deepfake detection market is heading.
How Deepfakes Work
Parya Lotfi and Mark Evenblij are both engineers trained at the Technical University of Delft and are the founders of the Dutch deepfake detection startup DuckDuckGoose. Lotfi once had her identity stolen for an online scam, motivating her to found her startup.
“I only have one picture on LinkedIn and maybe one picture on Instagram,” she explains. “They took my LinkedIn picture and pretended to be me. What if they now also applied deepfake technology? That, for me, was the moment I realized this needs to be stopped.”
The advancement of deep learning technologies has made deepfakes increasingly lifelike. This is largely due to the use of generative adversarial networks (GANs), where two algorithms are pitted against each other to create and identify inconsistencies in deepfakes.
Here's how it works: The first component, called the "encoder," generates synthetic data, which is then used to train the second component, known as the "decoder," to distinguish between real and fake media. Through this process, deepfakes become incredibly difficult to distinguish from genuine media, whether by human observation or AI-powered detection systems.
Surprisingly, only a small media sample is needed to achieve these convincing results.
“We should realize that in this conversation we're having, this video call, we are both gathering enough data to make an excellent deepfake of each other.,” Evenblij says. “You have enough data for me to fake my voice, fake my face.”

The Dangers of Deepfake Technology
Deepfake technologies bring a lot of risks ranging from synthetic child pornography to financial fraud attempts, cybersecurity breaches, and even the impersonation of high-profile individuals, like CEOs, politicians, and celebrities, in live video calls or television. There are lots of use cases that should make us skeptical of what we see on our screens.
“The deepfake is a daughter of disinformation,” explains Dr. Bernd Pichlmayer, the former Cyber Security Advisor to the Federal Chancellor of Austria and a member of the European Cyber Security Challenge steering committee.
Deepfakes, he continues, create insecurity by influencing information landscapes, and are even becoming integral to modern hybrid warfare strategies on a national level. In other words, before you could believe what you see, like an image of a tank crossing into another country. Now, it’s hard to be certain of whether or not that image is real, but it might nonetheless spark a reaction, and in the worst case, start a war.
Regulating Deepfake Technology
Despite these evident dangers, Dr. Alexander Godulla, a professor of empirical communication and media research at the University of Leipzig as well as a member of the university’s Deepfake Project, warns that humans are often negatively biased towards new technologies.
“We tend to miss opportunities by focusing solely on risks,” he claims.
For example, in the entertainment industry, deepfakes are used to digitally alter the appearance of actors and to perform stunts without risking physical harm. Additionally, they provide opportunities for individuals who are unable to speak or require translation services to communicate effectively online.
Rather than advocating for a blanket ban on all deepfakes, the priority should thus be on identifying and mitigating harmful instances while allowing for legitimate uses. Naturally, this is easier said than done.
Perhaps regulation is the answer. At least that’s what about 400 AI experts demanded when they signed an open letter, urging for stricter laws governing deepfake technology in February 2024.
How to Respond to Deepfake Technology
Every year, online fake news costs us around $78 billion globally.
“It's a problem affecting multiple layers of our society," Lotfi says about deepfake detection. “From the technological world to the legislative world, it requires a multidisciplinary approach to resolve.”
Godulla agrees that a purely legal approach wouldn't be ideal.
“In the social democracy that is Germany, there is a right to freedom of expression, guaranteed by the Basic Law [the Constitution of the Federal Republic of Germany],” he says. “We also have freedom of art, guaranteed by the Basic Law.”
He adds that, beyond the challenges of creating laws, enforcing them is another issue.
“Even if we regulate things,” he says “that doesn’t mean people won’t continue to do what’s prohibited.”
What’s certain is that, especially when legal lines get blurry, people need to be aware of deepfakes and actively look out for them. But how do you do that? Godulla advocates for proper education, traditional research methods like source verification, and media literacy.
Nevertheless, Pichlmayer says that awareness alone isn't enough. Government security systems need to be overhauled to include cybersecurity and deepfake detection technologies as essential competencies. Lofti and Evenblij also emphasize the crucial role of technology in detecting deepfakes, despite the importance of regulation and awareness.
“Even a poorly made deepfake can be convincing if presented at the right time, in the right context, and targeting the right emotions,” says Lotfi. “Context matters, and we often believe what we want to believe.
How Technology Can Detect Deepfakes
There are several ways technology can detect deepfakes. Lotfi explains that “reality is extremely complex, and deepfakes can never fully capture it; they always leave their fingerprints behind.”
That said, as Godulla points out, deepfake detection technologies face a tortoise and the hare issue, struggling to keep pace with the rapidly improving quality of deepfakes. Despite this, various AI-powered deepfake detection methods for visual and audiovisual content are being developed, next to traditional identity verification (IDV) and content certification tools. The consensus from experts in this field is that these technologies will undoubtedly play a significant role in the tech landscape of tomorrow.
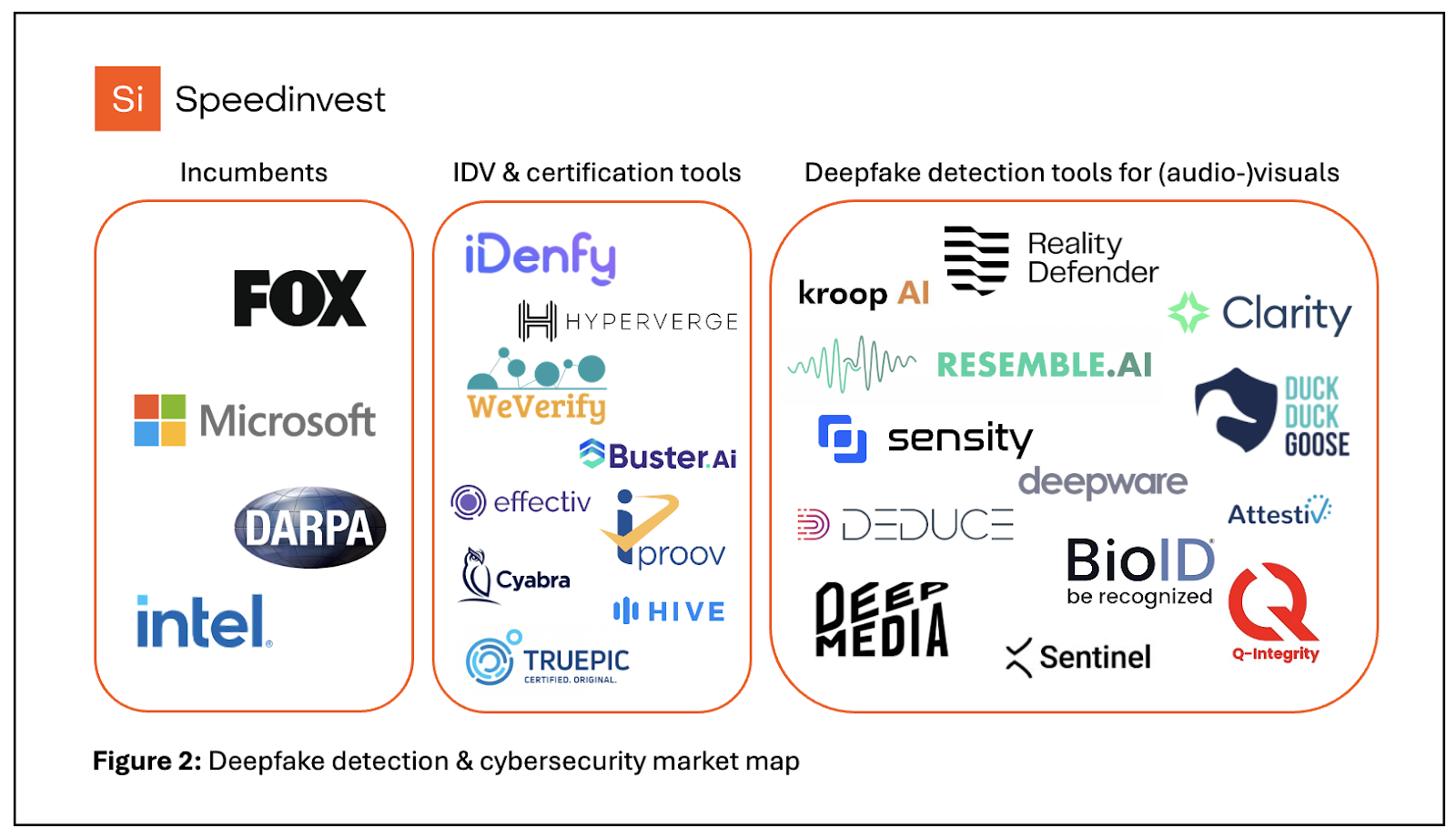
Key Considerations for Enhancing AI Deepfake Detection Technologies
To enhance the effectiveness of AI deepfake detection technologies, implementing pre-processing techniques is crucial. These techniques are employed to prepare the (audio-)visual data for analysis by extracting unique statistical aspects, such as facial features, and refining and adjusting the model accordingly. Then, deep neural networks are utilized for detecting fake images, while deep convolutional neural networks (CNN) and recursive neural networks (RNN) are employed for spotting fake videos.
The following models are designed to identify various characteristics, including:
- Physiological and biological features like blinking, eye movements, and heartbeat.
- Speech-face interaction mismatches.
- Temporal and spatial disparities between video frames.
Additionally, there are numerous factors that could potentially undermine the reliability and applicability of detection models, which we need to take into account. These include issues such as racial biases arising from a lack of diversity in the training data samples, as well as reduced accuracy levels due to inadequate quality in the training data, something Lofti and Evenblij emphasized. On top of that,, sophisticated adversarial attacks pose a significant threat to circumvent CNN technologies.
Lotfi asserts that their detection models accurately identify deepfakes in over 90% of cases. They aim to address the opacity sometimes associated with deepfake detection models by providing clients with detailed explanations for classifying media as deepfakes.
However, there is still room for improvement in the realm of detection technologies, as deepfakes continue to evolve. As a result, Lofti and Evenblij focus on fostering long-term relationships with customers, positioning themselves as trusted technology partners rather than mere vendors of AI technology.
Pichlmayer suggests also exploring alternative technologies, such as media signatures or e-certificates. Additionally, Godulla proposes that leveraging proprietary, certified hardware could prove beneficial. Determining which detection method to implement in specific use cases remains a task as the threat of deepfakes continues to grow in prominence and urgency.
All four experts concur that deepfake detection is likely to first become a focal point for social media platforms, particularly concerning content featuring significant individuals or events, which may be subject to automatic verification, possibly even before uploading.
However, the scope of these technologies extends far beyond social media. Globally, the fake image detection market is projected to grow at a CAGR of over 41% in just the next five years. Anticipating this growth, experts anticipate collaboration of detection technology providers with various stakeholders in the media, government, and business sectors to develop tailored solutions blended with traditional or manual detection methods.
Deepfake Detection Technologies Are Here To Stay
Deepfakes are not only here to stay but are also rapidly evolving, challenging the boundaries of detection technologies, and driving changes in regulation, education, and cybersecurity infrastructures.
Godulla acknowledges that the technical competence of people is low when it comes to the topic of deepfakes.
“The worries, on the other hand, are very large,” he says.“This creates a lot of uncertainty, which leads to conversations like this one: everyone is looking for guidance, for applications, for business models, for protection.
After diving deep into the realm of deepfake detection, here's what we've learned as investors:
- Deepfake threats impact various industries and necessitate a multidisciplinary approach for resolution, encompassing detection technologies (both software and hardware), regulatory frameworks (both local and international), as well as awareness and education initiatives.
- Deepfakes present opportunities for creatives, translators, educators, and more. Investors should keep an eye out for emerging business models in those sectors as well.
- AI-powered deepfake detection and content certification technologies are continually evolving to meet the increasing demand for protection. While it's unclear which technology or company will ultimately lead the field, one thing is for sure: these technologies are here to stay.
As VC investors, we are in a unique position to drive forward disruptive technologies, despite significant uncertainty. Our SaaS & Infrastructure team at Speedinvest has dedicated substantial time to exploring (cyber-)security as part of our investment thesis.
If you're a founder working in this space, don't hesitate to reach out to our team at saas@speedinvest.com. You can also find our team on LinkedIn: Markus Lang, Frederik Hagenauer, Florian Obst, Audrey Handem, and Anne Roellgen.
Want more updates on our portfolio? Sign up for our monthly newsletter and follow us on LinkedIn.